Random Forests®
Breiman and Cutler's Random Forests
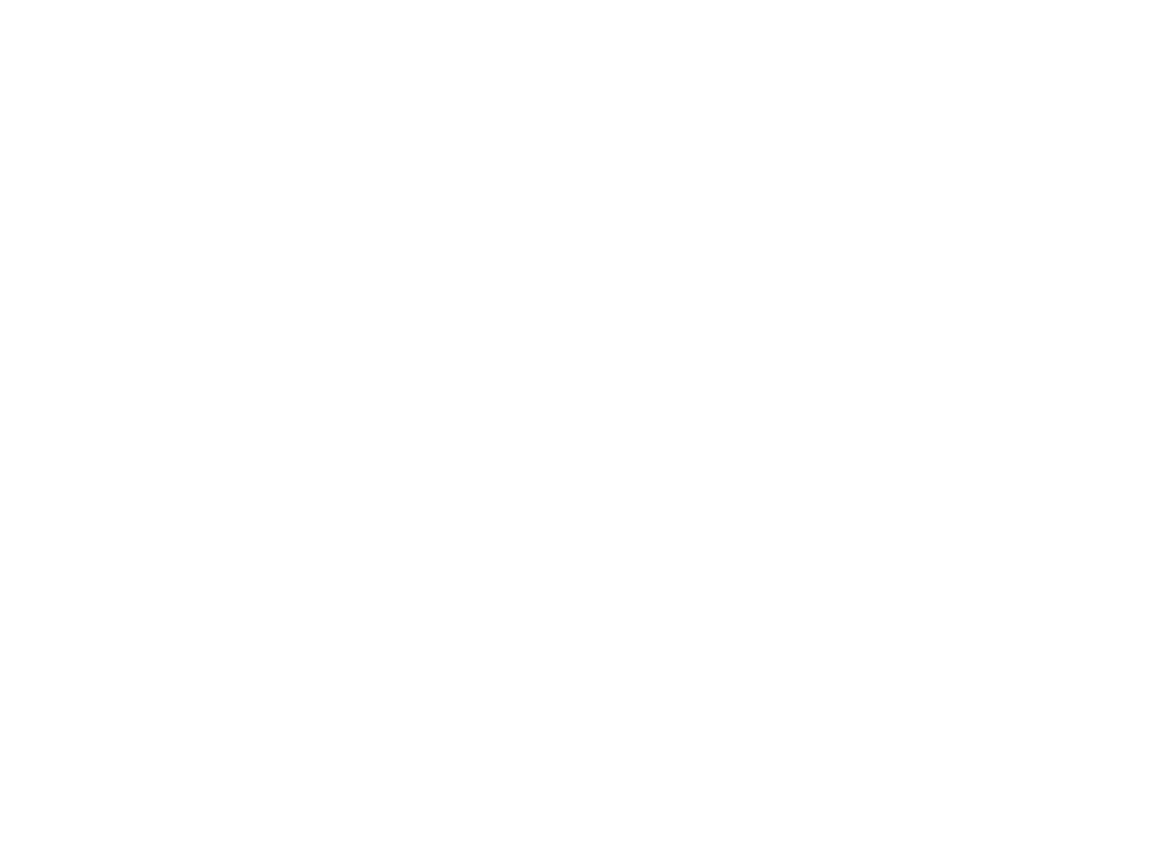
Based on a collection of Classification & Regression Trees (CART®), Random Forests® modeling engine sums the predictions made from each CART tree to determine the overall prediction of the forest, while ensuring the decision trees are not influenced by one another.
For those new to Random Forests, it is a powerful ensemble technique developed by Leo Breiman and Adele Cutler at the University of California, Berkeley, and is favored by many predictive modeling practitioners. The deceptive simplicity of the algorithm builds hundreds of independent trees and employs lots of sampling from both observations and variables.
Random Forests’ unique ability to evaluate unbiased model performance based on the out-of-bag data removes the need to have a separate testing/validation sample. This immediately positions Random Forests as the top predictive modeling tool in the wide data applications where the number of variables exceeds, often many times over, the number of available observations.
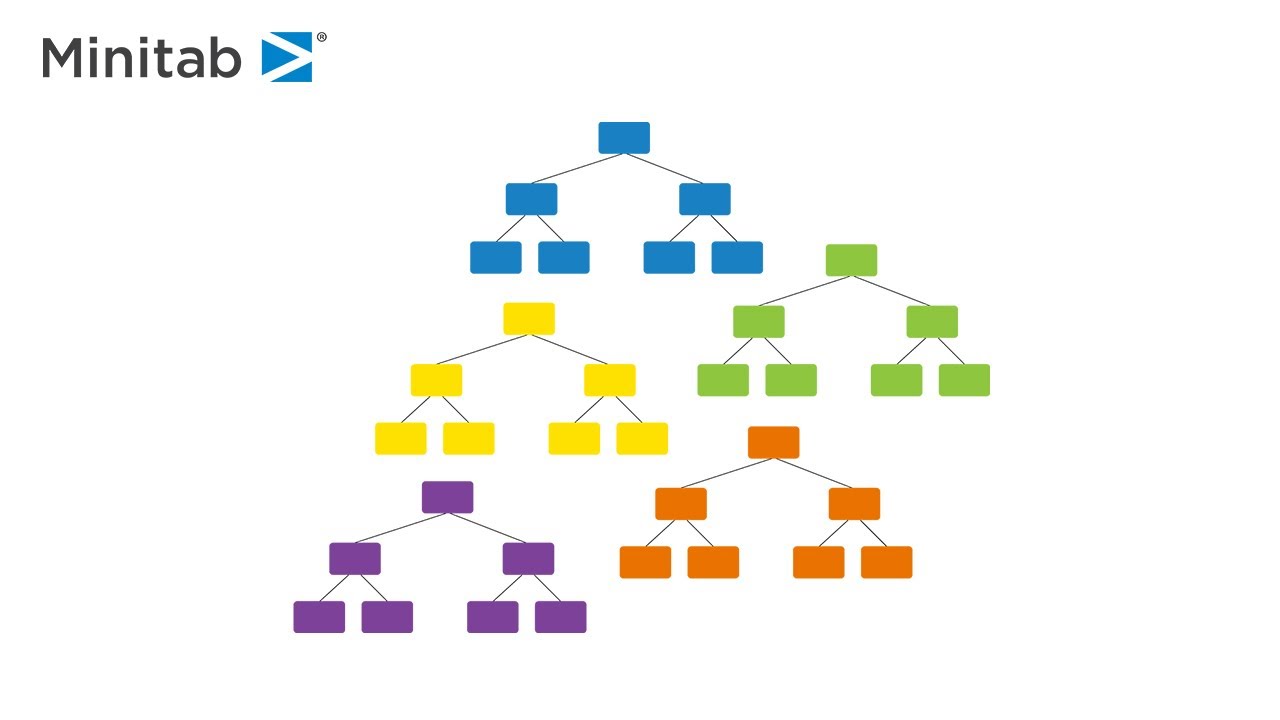
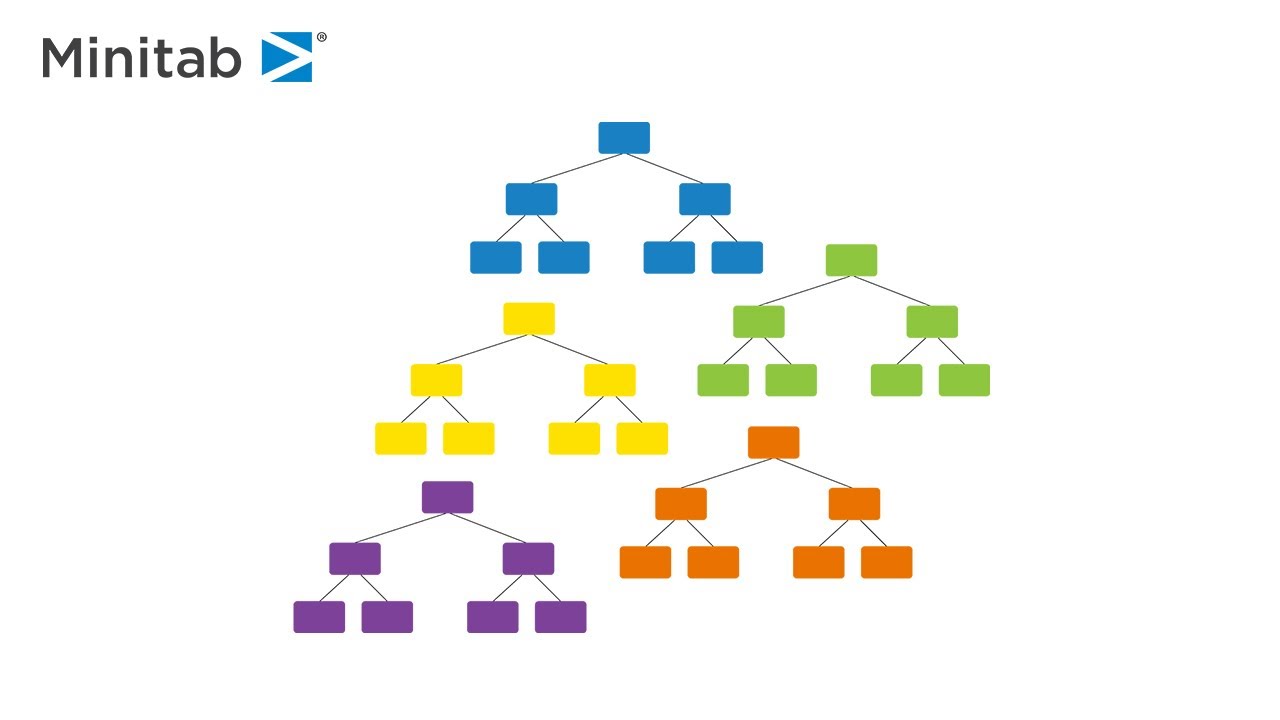
Accountability
Random Forests has a unique ability to leverage every record in your dataset without the dangers of overfitting. This is especially important for small (in terms of observations) datasets, where each record may contribute something valuable. Random Forests will make sure that all records have been accounted for in your models and no single insight has been lost.
Robust Variable Importance
Random Forests utilizes novel techniques to rank predictors according to their importance. This is convenient when the data includes thousands, tens or even hundreds of thousands of variables or predictors, which is well beyond the reach of conventional regression and classification tools. Random Forest can handle such extreme situations and report back which variables to use in follow-up research. Multiple rounds of sampling will add robustness and quality to these insights.
Interested in Trying the Most Popular Modeling Engine, Random Forests?
Minitab's Tree-Based Methods
Whether you're just getting started or looking to take your predictive analytics capabilities to the next level, Minitab's tree-based modeling engines have the power you need.
Learn more about all of Minitab's predictive analytics solutions
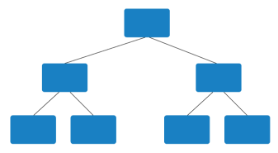
CART®
The ultimate classification tree algorithm that revolutionized advanced analytics and inaugurated the current era of data science.
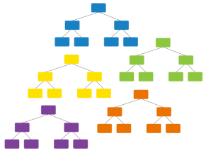
Random Forests®
The power to leverage multiple alternative analyses, randomization strategies, and ensemble learning in one convenient place.
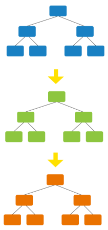
TreeNet®
The most flexible and powerful machine learning tool that is capable of consistently generating extremely accurate models.